Vinepredict – AI-Driven Powdery and Downy Mildew Forecasting Models
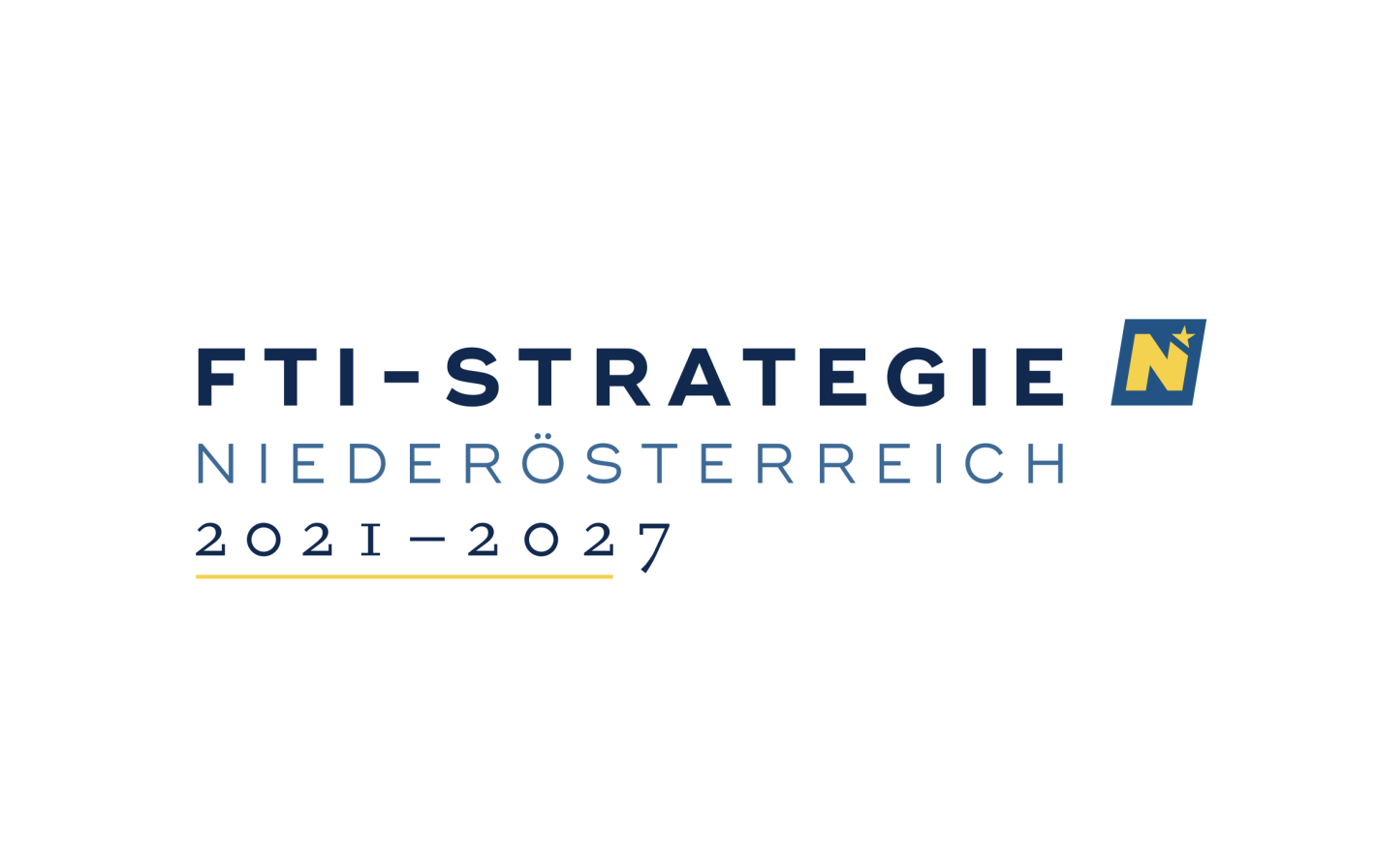
Lead partner:
Wein & Obst Klosterneuburg RTD - Einrichtung mit eigener Rechtspersönlichkeit an der höheren Bundeslehranstalt und Bundesamt für Wein- und Obstbau Klosterneuburg
Scientific management:
Markus Redl
Additional participating institutions:
Universität für Bodenkultur Wien (BOKU) - UFT Tulln
Universität für Weiterbildung Krems (Donau-Universität Krems)
Christian Hofmann Unternehmensberatung GmbH
Field(s) of action:
Digitalization, intelligent production and materials
Environment, climate and ressources
Scientific discipline(s):
4019 - Sonstige Land- und Forstwirtschaft, Fischerei (100 %)
Funding tool: Applied research projects
Project-ID: FTI23-A-001
Project start: 01. Jänner 2025
Project end: 31. Dezember 2027
Runtime: 36 months / ongoing
Funding amount: € 349.433,00
Brief summary:
The primary diseases in viticulture are downy mildew (Plasmopara viticola) and powdery mildew (Erysiphe necator). To control both pathogens, winegrowers must regularly spray fungicides and use forecast models for timing of applications. Prognosis for disease development is typically based on large-scale weather data as input parameters, but these do not reflect small-scale differences in disease appearance. This often leads to unnecessary applications in many vineyards. Furthermore, weather parameters are usually measured outside the vineyards, which does not reflect the actual microclimate in the canopy. It results in disease predictions that are not adapted to the local conditions in a vineyard. The objective of our project is to train artificial neural network models, namely sequence models, to predict downy and powdery mildew. We use not only site-specific disease level and weather parameters as training data but also the parameters vine development, vine management and vegetation cover. The training data consists of values gathered for 5 years before the start of the project and newly collected data in 15 vineyards. Unlike sensors used in other studies, this approach records leaf wetness on both the lower and upper sides of the leaf, which is an important parameter for both pathogens. Our models’ forecast will be tailored to the specific location and will predict disease progression up to 10 days in advance. By adapting forecasts to the location, fungicide use can be reduced. The output of the models can provide valuable information for winegrowers on when to apply fungicides, thereby contributing to a sustainable and environmentally friendly use of fungicides.
Keywords:
AI prediction models, plant protection, powdery mildew, downy mildew, forecarst models, viticulture