SADAA - Sensor-based anomaly detection – as a requirement in autonomous arable farming
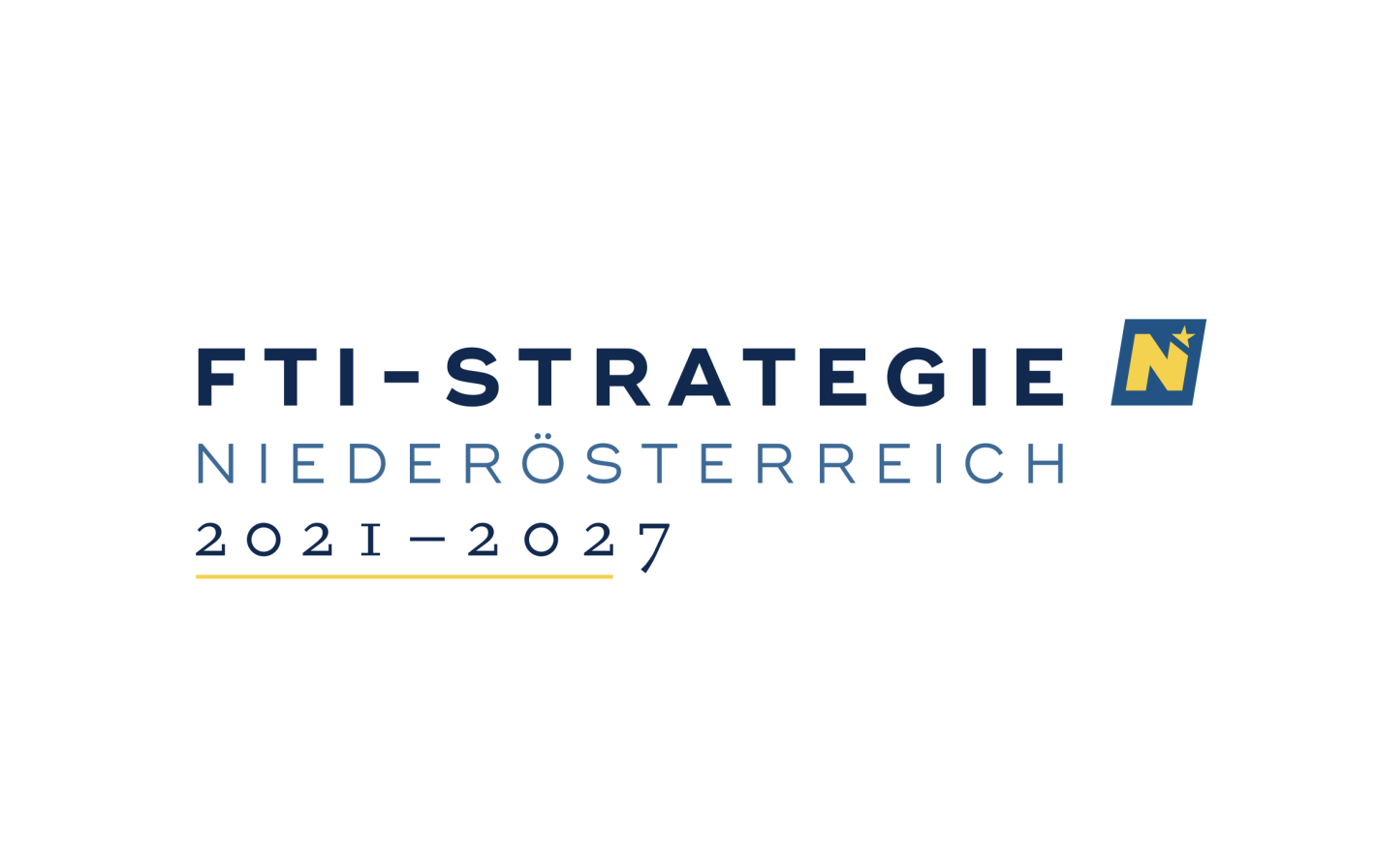
Lead partner:
Josephinum Research
Scientific management:
Peter Riegler-Nurscher
Additional participating institutions:
Fachhochschule Wiener Neustadt
Field(s) of action:
Digitalization, intelligent production and materials
Scientific discipline(s):
4011 - Ackerbau, Gartenbau (20 %)
1020 - Informatik (60 %)
2020 - Elektrotechnik, Elektronik, Informationstechnik (20 %)
2030 - Maschinenbau
Funding tool: Basic research projects
Project-ID: FTI23-G-009
Project start: 01. März 2024
Project end: 28. Februar 2027
Runtime: 36 months / ongoing
Funding amount: € 360.000,00
Brief summary:
Agriculture faces many challenges, including labor shortages and pressure to develop more sustainable practices. Meeting these challenges will require the use of autonomous systems. Agricultural robots can be used in a variety of areas for automation, including crop protection, harvesting, weed control and monitoring.
Agricultural field operations are characterized by complexity and a wide variety of scenarios. Until now, the demanding task of process monitoring has been performed exclusively by humans. First available autonomous systems for agriculture focus on process control and these systems largely lack the capabilities to automatically monitor processes. However, comprehensive process monitoring provides the indispensable foundation for full autonomy.
Especially in mechanical weed control and mowing, a wide variety of failure or anomaly scenarios can occur, such as blockages, tool damage, faulty tool guidance, etc. These are directly visible or indirectly via the work quality of the machine.
To detect anomalies and errors in the process, a wide variety of sensor technologies, such as camera systems, lidar, radar, current measurement, etc., can be used. We are proposing three alternative approaches to the multimodal anomaly detection problem for broad applicability in agriculture: A system which individually checks for anomalies in different data domains with separate deep neural network models and fuses them into a single anomaly score later. The second alternative aims to process multimodal data in a single deep neural network, using latest advances in deep learning based anomaly detection approaches and multimodal fusion techniques. The third final model is an end-to-end to deep network design, which learns both representations and anomaly scores directly from the data. We also plan to collect an open anomaly detection dataset targeted for agricultural machinery, which contains data from different modalities.
Keywords:
digitalisation, robotics, agriculture, big data, internet of things, aritficial intelligence, machine learning, sensor systems, anomaly detection